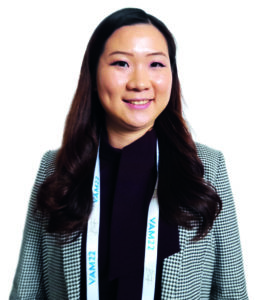
Artificial intelligence (AI) models can be developed to predict the risk of postoperative complications after endovascular aneurysm repair (EVAR) with “high accuracy,” research presented at this year’s Society for Vascular Surgery (SVS) Vascular Annual Meeting (VAM 2022; 15–18 June, Boston, USA) demonstrated.
“Complications after EVAR can be fatal,” Becky Long (Central Michigan University, Saginaw, USA) informed attendees, noting in her presentation that patient follow-up for surveillance imaging is becoming more challenging as fewer patients are seen, particularly after the first year.
The aim of Long’s study was to develop an AI model to predict the complication probability of individual patients to better identify those needing more intensive surveillance. The research involved collecting preoperative computed tomography angiography (CTA) 3D reconstruction images of abdominal aortic aneurysm (AAA) from 273 patients who underwent EVAR from 2011‒2020.
Of these patients, 48 had postoperative complications including endoleak, AAA rupture, graft limb occlusion, renal artery occlusion, neck dilation, pelvic ischaemia, and graft migration.
Using a deep convolutional neural network model, the researchers utilised the 3D CT images to predict the risk of complications after EVAR. The model was built with Tensorflow software and run on the Google Colab Platform.
A training subset of 40 randomly selected patients with complications and 189 without were used to train the AI model. Eight positive and 36 negative cases tested its performance and prediction accuracy. Data downsampling was used to alleviate data imbalance and data augmentation methodology to further boost the model’s performance.
Long’s presentation reported that successful training was completed on the 229 cases in the training set and then applied to predict the complication probability of each individual in the held-out performance testing cases.
The model provides a complication sensitivity of 100% and identified all the patients who later developed complications after EVAR. Of 36 patients without complications, 16 (44%) were falsely predicted to develop complications.
The results, therefore, demonstrated excellent sensitivity for identifying patients who would benefit from more stringent surveillance and also alleviate the need for surveillance in 56% of patients unlikely to develop complications, the presentation showed.
This, Long concluded, demonstrates that AI models can be developed to predict the risk of postoperative complications with high accuracy.
“Compared to existing methods, the model developed in this study did not require any expert-annotated data but only the AAA CTA images as inputs,” Long’s presentation showed. “This model can play an assistive role in identifying all patients at high risk for post- EVAR complications and the need for greater compliance in surveillance.”